Data science and data analytics are two of the fastest growing fields of study in the world. But what exactly is the difference between the two? Is one more powerful than the other? In this blog post, we will explore the differences between these two powerful disciplines, and uncover which one has more potential for businesses and individuals. Read on to find out!
What is Data Science and Data Analytics?
Data science is a field of study that applies data-driven approaches to problems in order to produce actionable insights. Data analytics, on the other hand, is a process or set of techniques for turning raw data into meaningful information that can be used to make informed decisions.
The two fields differ in their approach to problem solving and their focus on data analysis. Data science typically relies heavily on statistics and machine learning while data analytics traditionally focuses more on business considerations such as user behavior and customer segmentation. However, both fields are often intertwined and employ common methods such as exploratory data analysis and hypothesis testing.
In order to produce accurate insights, data scientists need access to large amounts of relevant statistical data as well as knowledge about how computers work. They also need the ability to think critically about complex problems and understand how best to apply various analytical techniquesets. All together, these skills makedata science an attractive option for companies seeking innovative solutions to important business challenges.

Exploring the Differences Between Data Science and Data Analytics
There are a few key differences between data science and data analytics. Data science is all about the analysis of large data sets, while data analytics is about using that analysis to make decisions for business purposes.
One of the biggest benefits of data science is that it can help us better understand ourdata. By understanding it in greater detail, we can better identify patterns and relationships that we might not have noticed before. This helps us make more informed decisions when dealing with our data, which ultimately leads to improved businesses results.
Data analytics also offers businesses a number of advantages over traditional decision-making methods. For example, by using big data analytics techniques we can speed up the process of making decisions and improve our accuracy overall. In addition, Analytics allows us to quickly analyze trends and understand how customers are interacting with our products or services – giving us vital insights into how best to serve them in the future.
So although there are some key differences between these two disciplines, ultimately they both offer tremendous benefits for businesses across a variety of areas. It’s important to carefully consider what will be best suited for your specific needs before jumping into either one though – as there isn’t one “right” answer!

Examining the Impact of Data Science and Data Analytics on Businesses
Data Science and Data Analytics are two different types of data-related disciplines that can have a big impact on businesses.
Data Science is all about understanding the data and using that understanding to solve problems. Data Analytics is the process of turning data into actionable insights so that businesses can make better decisions.
Data Science can help businesses improve their decision-making processes, understand customer behavior, and optimize their operations. Data Analytics can help businesses find new opportunities, predict future trends, and create more effective marketing campaigns.
Both Data Science and Data Analytics have a lot of benefits for businesses. However, it’s important to choose the right tool for the job and to use it in the right way. If used improperly, data science and data analytics can have negative impacts on businesses.
So far, data science has had a bigger impact on business than data analytics. That’s likely because data science is a more recent discipline and has yet to be fully developed. However, as data analytics becomes more sophisticated, it’s likely that its impact on business will grow even more.

Leveraging the Benefits of Both Data Science and Data Analytics
Understanding the Fundamentals of Data Science and Data Analytics
Both data science and data analytics are important tools for understanding and manipulating data. However, they have different purposes and can be used in different ways. Data science is focused on understanding the data and using that understanding to develop models or algorithms. Data analytics, on the other hand, is focused on using the models or algorithms developed by data science to make decisions.
Data science is often used to improve the quality of data, make it more accessible, and enable faster analysis. Data analytics is often used to make decisions based on the analysis of data. For example, a company might use data analytics to decide which products to produce, how to market them, and where to invest money.
Both data science and data analytics have their own benefits and drawbacks. Understanding these fundamentals can help you to better leverage the benefits of each tool.
Exploring the Benefits of Each Field
Both data science and data analytics have many benefits that can be leveraged together to improve business operations. When practiced correctly, both disciplines can help identify patterns within vast amounts of data, develop models to predict future outcomes, and make better decisions. Data science focuses on the analysis and interpretation of data using a variety of algorithms and tools while data analytics applies the principles of statistics and machine learning to analyze large datasets. The following are four key advantages of using both data science and data analytics in your business:
- Improved Quality Assurance: With data science, you can use sophisticated analytical methods to ensure that your results are accurate. This helps you avoid making errors during the analysis process, which can lead to incorrect conclusions or worse yet, faulty actions.
- Increased Accuracy and Speed: With data analytics, you can quickly analyze large datasets to identify patterns and insights that would otherwise be difficult or impossible to find. This helps you make decisions more quickly and improves the quality of your business operations.
- Greater Flexibility: Data science allows you to create customized models that are tailored to your specific needs. This means that you can design solutions specific to your company’s unique situation, instead of relying on predefined models or algorithms.
- More Precise Results: When using data analytics,you can reduce the amount of subjective interpretation required in order to arrive at accurate results. By automating many of the analysis steps,you can ensure greater accuracy and consistency throughout your data analysis process.
Determining Where to Focus Your Resources
Both data science and data analytics offer distinct advantages for businesses. However, it can be difficult to determine where to focus your resources when it comes to data science vs. data analytics. In this article, we will explore the benefits of both fields and help you determine where to focus your efforts.
Data science is a process of extracting meaning from data using algorithms. Data analytics, on the other hand, is the application of analytics techniques to understand and make decisions based on data. While both fields offer a wealth of benefits, it is important to understand which toolset will best suit your needs.
Data science can help you find patterns in your data that you may not have otherwise noticed. By understanding the relationships between different pieces of data, you can develop insights that can improve your business.
Data analytics can help you make better decisions based on the data you have. By using analytics techniques, you can identify patterns and trends in your data that may not be apparent to you otherwise. This information can help you make informed decisions about your business.
Both data science and data analytics offer a wealth of benefits for businesses. However, it is important to understand which toolset will best suit your needs. By understanding the benefits of each field, you can make the most effective use of your data resources.
Maximizing Results Through Combined Strategies
Data science and data analytics are two complementary fields of study that can provide powerful insights into how to improve the performance of a business. However, it’s important to tailor which approach to use based on the specific problem you’re trying to solve.
If you want to identify patterns in large amounts of data, for instance, data science would be a better approach. Data analytics, on the other hand, can help you make sense of that information and use it to predict future outcomes.
By combining data science with data analysis techniques like machine learning, you can really unlock the power of both fields. This way, you get more accurate predictions and deeper insights about your data.
Applying Data Science and Data Analytics to Real-World Problems
As data science and data analytics continue to grow in popularity, businesses are beginning to realize the benefits of these two disciplines. Data science is focused on understanding and extracting meaning from data, while data analytics is the process of using that data to make decisions.
Data science can help businesses improve their understanding of their customers, their products, and their competition. It can also help businesses identify new opportunities and develop new strategies. Data analytics can help businesses make better decisions by providing them with the information they need to understand the data and make informed decisions.
Data science and data analytics are growing in popularity because they offer a number of benefits that are difficult to find elsewhere. They can help businesses improve their understanding of their customers, their products, and their competition. They can also help businesses identify new opportunities and develop new strategies.
Choosing the Right Tool for Your Business Needs
Understanding the Technical Differences Between Data Science and Data Analytics
When it comes to data analytics, there are two main tools in the toolbox: data science and data engineering. Both data science and data engineering can be used to address different business needs, but there are some key technical differences between them that should be taken into account before deciding which tool is right for your business.
Data Science focuses on using a combination of statistical techniques, machine learning methods, and assumptions about the problem to find insights. Data engineers build automated solutions using programming languages like Java or Python.
One important difference between these two fields is that data engineering generally deals with building programs or models instead of analyzing datasets. This means that it’s better suited for tasks such as automating analysis or pulling insights from large amounts of data.
Data engineering is also useful for tasks that don’t involve data science, such as designing scalable systems or figuring out how to best use existing programs. In contrast, data science is primarily used for discovering insights from datasets.
One final difference between these tools is the skillset needed to use them. Data engineers generally have more programming skills than data scientists, but both groups of professionals need a good understanding of statistics and machine learning in order to be effective.
Identifying Your Business Goals: How Does This Impact Choice of Tool?
Data science and data analytics are both fields that focus on understanding data to make better decisions. However, there are some key technical differences between the two fields that should be taken into consideration when deciding which tool is best for your business needs.
One of the main differences is that data science focuses on using algorithms anddata extraction techniques to analyze and understand large datasets. This can be used to identify patterns or insights that were not possible to see with simpler methods. Data analytics, in contrast, focuses more on using this informationto make informed decisions about how to manage or use the data.
Another important difference is that data science typically requires a much larger dataset than data analytics does. While a small dataset may be sufficient for analysis bydata mining techniques, it may not be enough for data science techniques. In addition, data sciencerequires a much more in-depth understanding of the data than does data analytics. This means thatdata scientists typically require more training and experience than databasicians do.
Finally, while both fields can be used to support decision making at any level ofthe organization from marketing to operations,data science is often seen as being more geared towardssupporting high-level decision making. This might involve using complexmodels or simulations to make predictions about future behavior or trends. Data analytics, on the other hand, ismore focused on providing immediate insights that can be used to improve decisionmaking at lower levels of the organization.
Is Combining Both Tools a Viable Option?
Data science and data analytics are two of the most popular tools in business today. They both have their own strengths and weaknesses, so it’s important to choose the right tool for the job.
Data science is all about understanding data and using that understanding to solve problems. Data analytics, on the other hand, is all about using data science techniques to make decisions.
If you’re only looking to use data science to understand your data, then you don’t need to combine it with data analytics. However, if you want to use data science to make decisions, then you need to combine it with data analytics.
There are a few reasons for this. First, data science is very effective at understanding your data but not as good at making decisions. Second, data analytics is good at making decisions but not as good at understanding your data. Finally, combining the two can help you achieve the best of both worlds.
So, if you’re looking to use data science to solve problems, then you should combine it with data analytics. If you’re looking to use data science to make decisions, then you should combine it with data analytics and use machine learning techniques to improve accuracy.
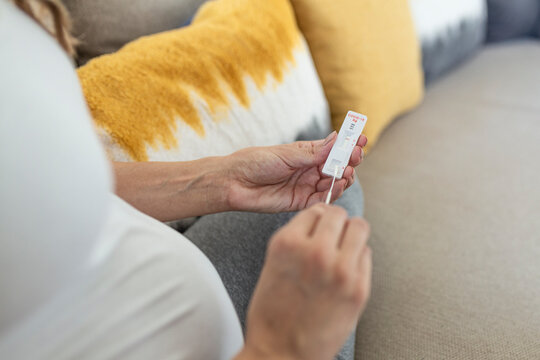
Common Pitfalls of Working with Data Science and Data Analytics
As data becomes increasingly important to businesses, it is essential that they allocate resources wisely when using data science and data analytics. While the two fields share some common goals, there are also key differences that must be taken into account when working with these tools. Here we explore the most important of these differences.

Understanding the Future of Data Science and Data Analytics
The future of data science and data analytics looks very promising. As technology advances and we become more aware of the power of data, it seems that the two disciplines will continue to grow in popularity. Data science focuses on the analysis and interpretation of data, while data analytics is all about using that information to improve business operations.
Data science has already demonstrated its ability to solve complex problems. For example, Facebook used data science to develop a model that can predict how people might react to different types of content. And as machine learning becomes more sophisticated,data scientists will be able to use this technology to identify patterns in datasets extremely quickly.
Meanwhile, data Analytics is also becoming increasingly important. Organizations are now realizing that they need access to real-time insights in order to make informed decisions. This is where big Data comes in – meaning the collection, management and analysis of lots of structured and unstructured data sources – such as social media posts, customer interactions and email logs. By harnessing these kinds of datasets, companies can better understand their customers and competitors alike.
So while there are some inevitable challenges ahead for both fields (for instance: ensuring that all the disparate information is analysed correctly), overall it appears that data science and DATA ANALYSIS will have a bright future together!
Data science and data analytics are powerful tools that can help businesses gain valuable insights into their operations. By understanding the differences between the two disciplines, businesses can make informed decisions about which tool is best suited for their needs. With the right approach, data science and data analytics can be used to solve real-world problems and help businesses reach their goals. With the ever-evolving landscape of data science and data analytics, it is important to stay up-to-date on the latest trends and developments in order to remain competitive. By leveraging the power of both data science and data analytics, businesses can unlock new opportunities for growth and success.
Frequently Asked Questions
Q.Who uses Data Science & Analytics?
A.Businesses use both to gain insights.
Q.What is the difference?
A.Data Science focuses on prediction, Analytics on analysis.
Q.How do they work together?
A.Data Science provides data for Analytics to interpret.
Q.What are the benefits?
A.Both provide valuable insights to inform decisions.
Q.How do I choose?
A.Consider your goals & resources to decide which is best.
Q.What if I don’t have the resources?
A.Start with Analytics & build up to Data Science when ready.